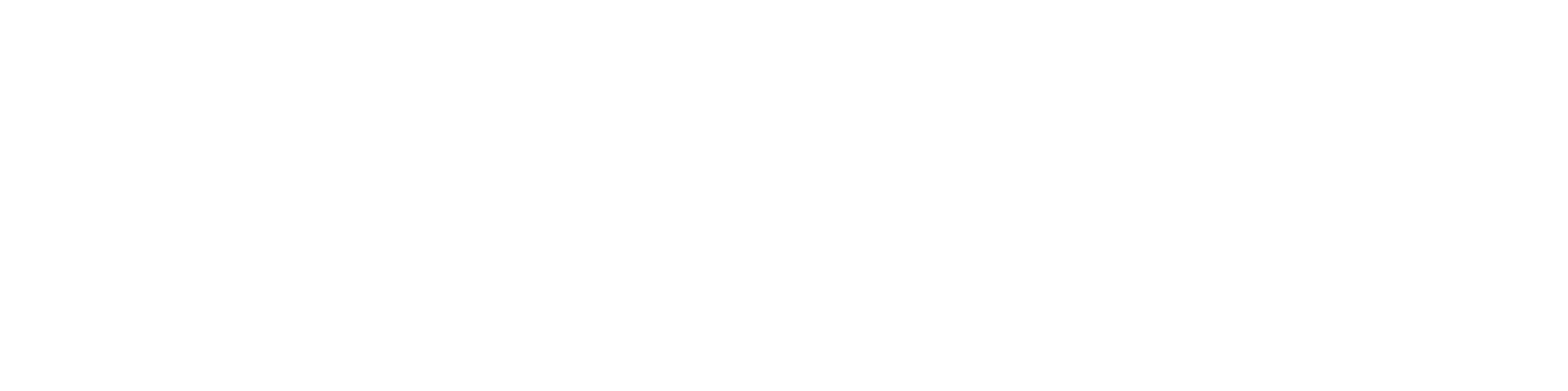
ReasonField Lab
VirtusLab Group Company
hello@reasonfieldlab.com
ReasonField Lab 2024, All Rights Reserved
A few months ago, Maciek Adamiak, the founder and former CEO of ReasonField Lab, appeared as a guest on the Nieliniowy podcast. During the episode, he shared his insights on discussing machine learning and provided tips on how to improve at it. The podcast is available in Polish, and you can find more information and links to the episode in this post.
However, we have an excerpt of the interview in English for non-Polish readers that you can find below. Enjoy!
Hello, nice to meet you. By the way, I am also the president of ReasonField Lab, a subsidiary of SoftwareMill. It's a long history of the creation of this company and the idea for it in general. It's a spin-off of SoftwareMil founded by me and several other software developers. Currently, we are a small part of a company with a research task. The idea for this company is mainly to conduct interesting research and participate in projects that involve machine learning and, at the same time, are related to various types of primary sciences. Of course, apart from that, you also need to sprinkle it with some commercial projects to fund it properly. I like to say that I want to look for projects that have a significant impact on the reality around me. And I'm looking for good projects that I feel are needed.
But is rerouting the river a good idea? No, probably not. I have my own private interests related to remote sensing - studying the Earth using satellite images. And these are the nature issues I am looking for in my work. I won't say there are many AI projects, although we are lucky to have them and continue them. From the first idea when we started a medical project to today when we are also working on a pro-ecological project, it can be implemented. But it's super tricky, I'll admit it.
Due to NDA agreements, I can only say it is a project with a solid scientific basis, and its basic assumption was to pass the FDA audit in the United States, and it managed to do so. It means that it is a medical device already in production. It was a company that had already dealt with this before and financed it. They had very good foundations in building this type of model and fantastic infrastructure. The research problem was known, and a group of specific experts had been gathered. We just had to come up with a clever solution to this problem. That is, what we at ReasonField Lab know best and what we like the most.
This phase of this, let's call it incubation, is what I love. And I wouldn't say I like meetings much. However, there are also intensive, inspiring meetings that aim to work out the problem and formulate hypotheses. At ReasonField Lab, we have this part of the whole process. But generally, when we start talking to our clients, we suggest meeting them about a week before doing any group concept. It's what we call workshops. During this week, we talk, review the literature, and wonder what the current state of knowledge on this topic is. This procedure has not disappointed me yet; since I have been working in this industry, the workshops actually work well. When people start talking to each other, it only starts to make sense, and then it becomes easier. For example, they develop a common vocabulary during such a meeting. Everyone knows it will be a very intense week, and they are prepared for it. Very often, when clients come to workshops, they have already reviewed the literature. It's the perfect situation. The workshops are super effective.
Obviously, money is an important issue here. However, at the very beginning, no matter who we incubate the project with, we must first enthuse them with the fascination that we have - so that these people have the same motivation. For example, I am very interested in scientific research. Knowing that I will have a workshop with someone and we will consider some super difficult problems, often in a domain that I have no idea about before, and that I have a lot to learn, I am already super motivated. I have the impression that people are getting enthused with this motivation, which is the most important thing, that people come and say, okay, such a person is coming, and now it will be demanding. He won't give up here; even though we are his clients, he will demand a lot. Additionally, we know that this is the week for which the rate is appropriate. Even though it is not excessive, people feel this is the week they invest. And I'm happy that everyone is preparing very well as I think I have to prepare even better. It's a spiral of preparation. And then we have a good discussion. I once had a fantastic workshop with a specialist in his discipline, also in the field of medical diagnostics. This man spread his passion for how he talked, so well prepared that it was a powerful discussion. They had to force us out of the room. But a good thing came out of it.
At the very beginning, we start typical business work with the client; we talk about the basic conditions. Then, when I come to the workshops, I divide them into appropriate sections, depending on the type of client. If this is an approach in which it is not known whether ML is a good option, the workshop begins with a conversation about what machine learning is, how it differs from the other approaches, and whether the client actually needs it. Sometimes, they don't, and it's our responsibility to clearly state that because not everything needs to be solved with machine learning algorithms. And this is usually the first day. The second day is the day of asking questions. I plan a scenario for such a conversation to get as much information as possible from my potential client to understand the domain. I also prepare in advance - I review the literature and check what solutions are currently available. After such a conversation, I receive a dose of information that allows me to determine whether Proof of Concept is needed. Then, I present the client with the first possible solution, the simplest one. After the first part, I can say - okay, this is common knowledge; no Proof of Concept is needed. It will be a comfortable situation here. If you want to do a project, we can start immediately and set up a team. But sometimes, a Proof of Concept is needed as it is knowledge that has not been scientifically discussed often; the topic is not specified whether it is possible to implement - I clearly inform the client about it.
My approach is to explore the areas of activity of the company I am to work for, but also what kind of team I will be dealing with, what people can help me with this task, who is on board, who is already an expert, what knowledge is available, what is the history of this project and where did the idea come from? To cooperate well, you need to understand the person you work for and their intentions fully, why they want to implement this, and not something else. It's definitely easier to talk later, and you can quickly create a dictionary of terms. As you say, we all understand Proof of Concept as something different, so we need to establish this nomenclature. As for what PoC is for me, I approach it as a scientific process, so it poses a research problem and confirms hypotheses. So I tell the client the research problem, the hypotheses supporting it, what has been confirmed and rejected, and then what we do next with it.
Exactly, I think it's also essential to define what we will do and present the whole process. This is one of the ideas that we have during the workshops. On the first day, we actually talk about how we work and what we will do now. What does PoC mean? What does MVP mean? What does it mean that we work remotely? It is also a very, very interesting concept today. The most important thing is that this glossary of terms is known to make cooperating easier.
I think you have a very good point about this. The first thing is to immediately distinguish between classic development and machine learning development. We can predict approximately when the project will be completed. All the building blocks for a given solution are relatively known. However, in ML, apart from the fact that the blocks are known, the "car" is unknown - it is not known what the result is. It is the stage where you have to tell the story many times, and the easiest way is to use an example to be implemented. That's what these workshops are for me, after which I know more or less what we need to do, and I tell them what data we need. In fact, I may be marginalizing modeling or writing a machine learning model a bit because I'm just saying - this is the data we have, and this data has some noise; you also need to understand this noise; it will translate into further solutions. And it may not work anyway because I can't tell you. And this is, of course, the first question: how much data do I need? This is machine learning; this is research. I can plan a research project in such a way that, at some point, I will be able to say how much data we need. But not at the beginning; it often happens. The more honest one is about how things work, the better - there can be no surprises later. We do all PoCs and MVPs at a fixed price, as it is convenient to plan, and I think it's also super important. We don't say that one day we will finish this ML, but precisely determine, for example, half a year in which we will work. Then, after half a year, I will present the result to the client, but it can be something other than what they want. It is still research; we need to make them aware of it. But on the other hand, according to the fail-fast principle, let's do things quickly and talk a lot to know where everything is heading. Yes, I think honesty here is an essential part of teaching about machine learning.